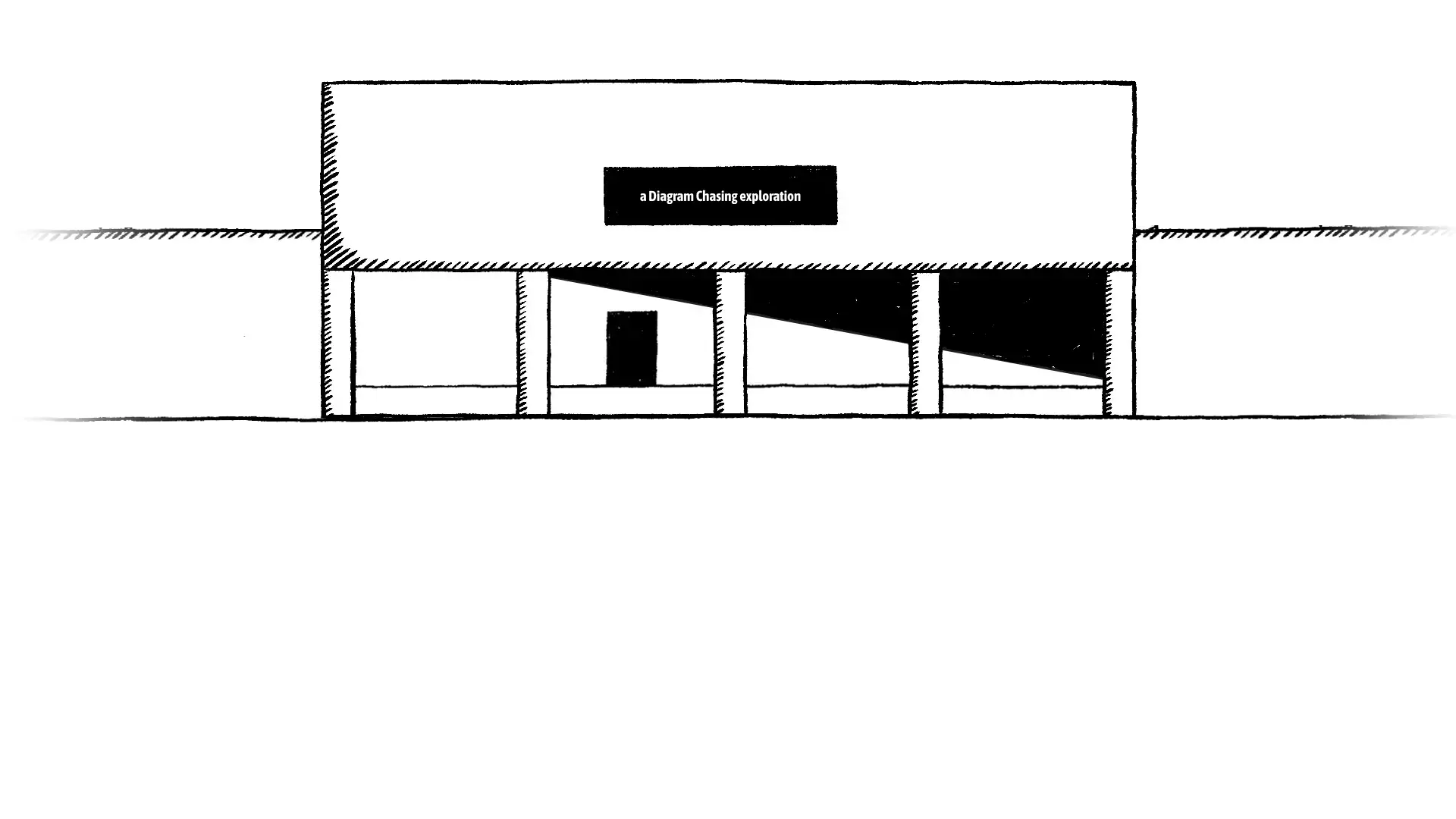
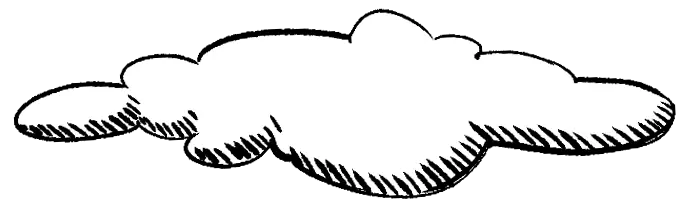
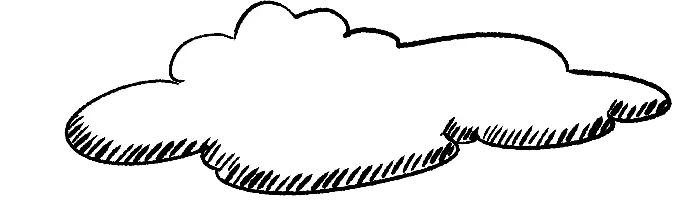
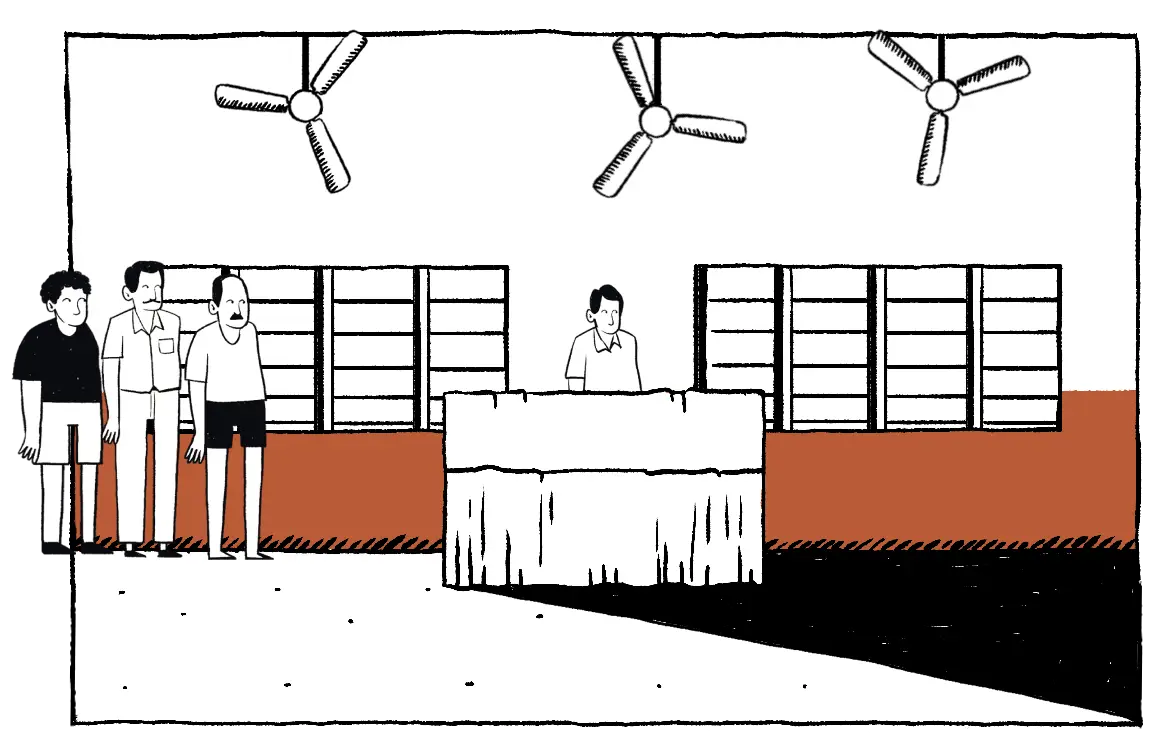
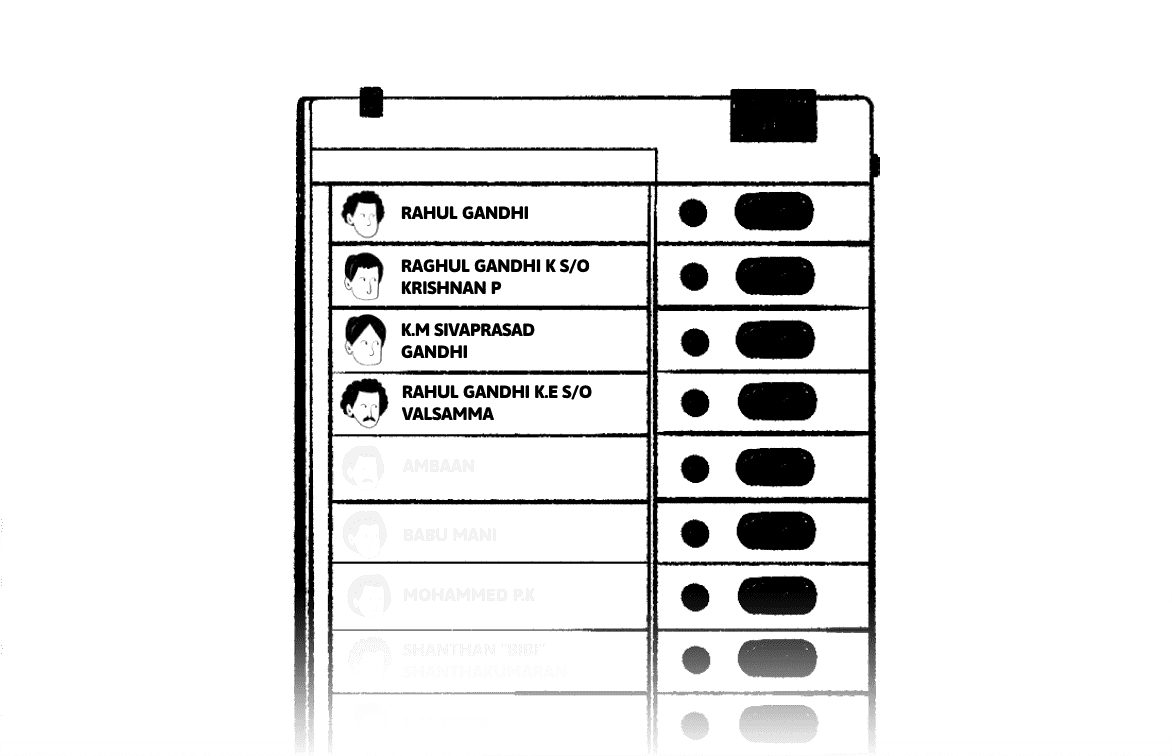
You step into the voting booth. The electronic voting machine hums in the converted classroom as the fan whirs overhead. Other voters behind you wait their turn. Your eyes search for the familiar symbol and name amid the rows of text.
This is a little weird. There's Rahul Gandhi that you recognize, but below sits another — Raghul Gandhi, with a different symbol. There's another man with the Gandhi last name right below. And one Rahul Gandhi KE more. That's a lot of Gandhis in one place.
In that election, 2196 voters chose Rahul Gandhi KE – a candidate whose campaign began at the last moment and politcal aspirations ended right after the polls. Whether these votes were because of confusion for the other Rahul Gandhi, or real conviction, is unclear.
In 2014, before photos of the candidates were put on EVMs, voters of a constituency in Chhattishgarh were faced between the choice of the BJP candidate Chandu Lal Sahu and 11 other Chandu Lal Sahus. All spelt almost all the same. Symbols, names, but no photos.
The real Chandu still won, but would have gotten at least 30,000 more votes without his duplicates in the running.
This doesn't just happen to a single party. Between 1960 to 2024, there are over 8000 candidates who share very similar names with their rivals – close enough to give careful voters pause, and perhaps misdirect the less aware into voting for namesakes who would disappear after election day.
Votes in a name
Namesakes in Indian Elections and How to Find Them
By Aman Bhargava
Art by Reechik Banerjee
India never really stops voting. At any given time, you’ll probably find some state gearing up for polls, managing an electorate that spans from city professionals to farmers across 28 states and dozens of languages.
To handle this diversity, the system relies heavily on symbols - INC’s hand, BJP’s lotus, AAP’s broom. These visual markers help voters navigate ballots that appear in multiple languages and help unify the message in a country where illiteracy is still high. While party candidates carry these established symbols, independents choose from Election Commission-approved ones, which are usually common objects like pressure cookers or ceiling fans.
But here’s where it gets interesting. While the system carefully prevents any confusion with party symbols, names are something of a blind spot. No two parties can share a lotus or a hand, but almost nothing stops multiple candidates from having nearly identical names. Until recently, the situation was exacerbated by the fact that the choices only appeared to voters as the names and symbols (which could also be alike) and no photographs of the people they were voting for. That changed less than a decade ago. Even so, sometimes, voters can find themselves doing double-takes at the ballot box, faced with candidates whose names are suspiciously similar to major contenders.
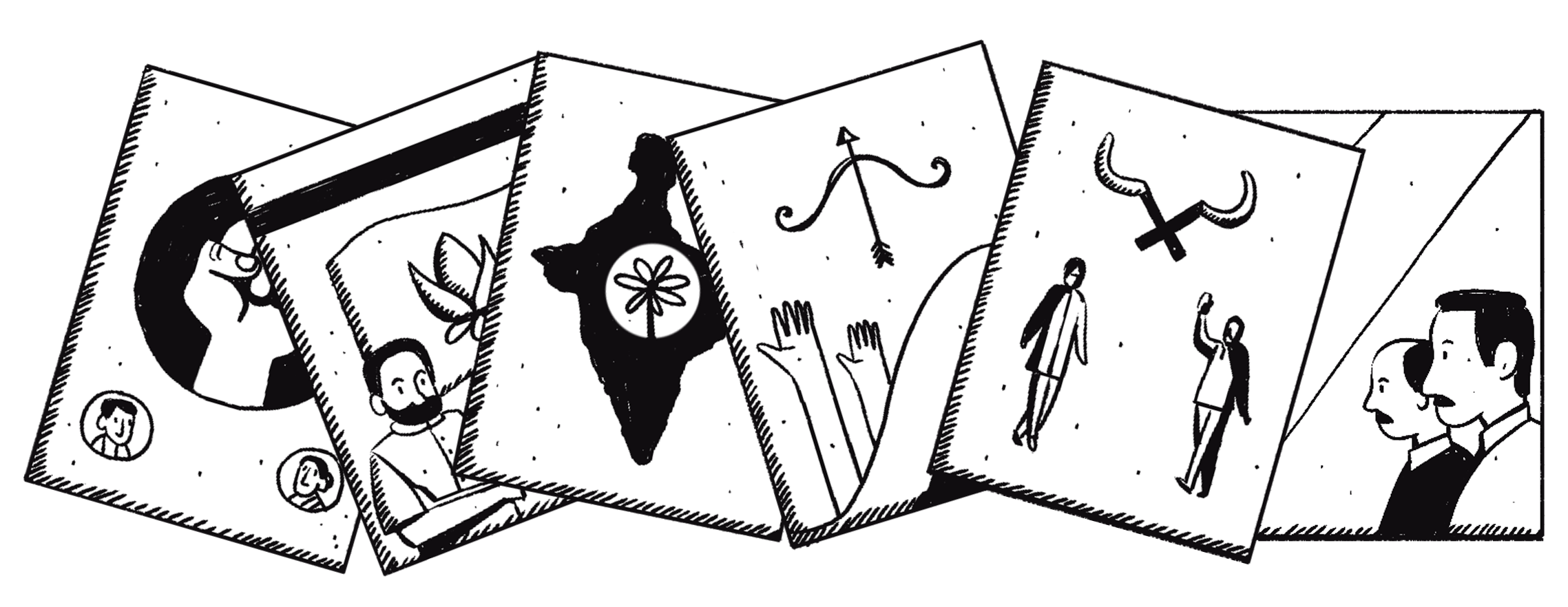
That list is quite long. In their constituencies, names like Alimineti Uma Madhava Reddy faced off with Alimineti Madhava Reddy, Raghubir Singh appeared on the same ballot as Raghvir Singh, H.D. Kumaraswamy faced D. Kumaraswamy, S.V. Ramani competed with S. Veeramani, Virender Singh and Virandar Singh, a specific Mohan Lal Badoli against a more ambiguous Mohan Lal, Rajendran A. versus Rajendaran B… you get the idea.
If you’re thinking, ‘Well of course these are confusing to me, I just heard of them right now’, that’s sort of the point. Unless you’re getting the same coverage as major candidates that generally dominate the headlines, a part of your electorate may be just as clueless if your campaign has limited reach. And that’s precisely what makes this potentially effective — it doesn’t need to fool everyone, just enough voters in what might be a tight race.
It’s of course unlikely that most of these namesakes could effectively sway an entire electorate, and some cases might be genuine coincidence — after all, common names exist. But it is an interesting exercise to find where all such cases appear and just how similar these names can get.
Name games
The Rahul and Raghul case, while high-profile, is less interesting precisely because it’s so visible. Voters are unlikely to be confused by such a well-known example. Most voters first see their local candidate’s written name at the EVM, where they must match what they’ve heard to what they see, in whichever script or language that may be. The more interesting cases lie in smaller constituencies and lesser-known candidates, where similar names have a greater impact.
The numbers are big, though. Between 1960 and 2023, across general and state elections, we’re examining approximately individual names, so I took some calls on how I filter it to a smaller, more relevant pool. After cleaning the data, the people in each constituency were divided into two groups — candidates who might face a namesake and the rest, for whom I had to figure out if they might be a namesake.
Name similarity can be measured in two ways: look-alike and sound-alike comparisons. To understand potential voter confusion, I needed ways to capture as much name similarity as possible (ideally, both visual and phonetic, but for this story, only visual).
The usual approaches to measuring similarity proved inadequate on their own. Levenshtein distance, which counts character edits, misses structural patterns in names. Dice’s letter-pair matching can stumble when similar names have different arrangements. Even the Jaro-Winkler algorithm, typically reliable for ordered names, struggles with variations of name order on ballots and spelling differences. Another, BI-SIM, offers a solution by analysing sequential letter pairs while maintaining word order, which can capture the flow of names much better, somewhat avoiding the shortcomings of simple letter-matching.
Have a look at a sample of the matches and how each of these algorithms scores them.
Differences in scoring
State | Reference Name | Similar Name | JW¹ | BI-SIM¹ | Dice¹ |
---|---|---|---|---|---|
Assam | Prodip Hazarika | Pradip Hazarika | 81.3% | 86.7% | 85.7% |
Bihar | Devandra Prasad Yadav | Devendra Prasad Yadav | 83.5% | 90.5% | 80% |
Gujarat | Rajeshbhai Chimanbhai Vasava | Ramanbhai Chimanbhai Vasava | 77.0% | 82.1% | 64.2% |
Madhya Pradesh | Mahendra Singh Bhadoriya | Devendra Singh Bhadoriya | 75.0% | 81.2% | 87% |
Tamil Nadu | Nageswari Thirumathi.n. | Maheswari Thirumathi .a. | 76.9% | 84.1% | 66.7% |
Uttar Pradesh | Dr. Narendra Kumar Singh Gaur | Narendra Kumar Singh Yadava | 70.8% | 78.6% | 71.7% |
They all do well in most cases, some are more eager to falsely match names than others, and some miss the obvious extent of similarity. So instead of relying on a single algorithm, I used all of them with different weights and only considered a pair of names to be a similar match if at least two algorithms agreed they were.
Let the algorithms vote too, if you will.
Even so, this process turned out to be incredibly sensitive to small changes in what we considered a threshold for matching. Names in India vary greatly by region, so after processing the entire dataset, I plotted what kind of names they identify and how it varied.
I think the above plot is fascinating because it shows you the range of names that each metric manages to capture, but we also see how certain states have names that are easier to capture. You’ll also notice that this is being shown to three decimal places. Since these values are sensitive to small changes, the difference between a 0.754
and 0.775
is not insignificant.
Each algorithm brings its bias - Jaro-Winkler tends toward much more optimistic matching (clustering toward higher similarity scores), BI-SIM remains conservative and grades similarity much more strictly, while Dice coefficient usually splits the difference. When two or more agree, we’re more likely to catch genuine name similarities rather than algorithmic quirks. Given the mixed salad that these ballots can be - last names before first names, fondly used terms (’bhaiya’, ’sahab’) popping up randomly, honorifics appearing wherever they may, or transliteration chaos switching between scripts, this is useful.
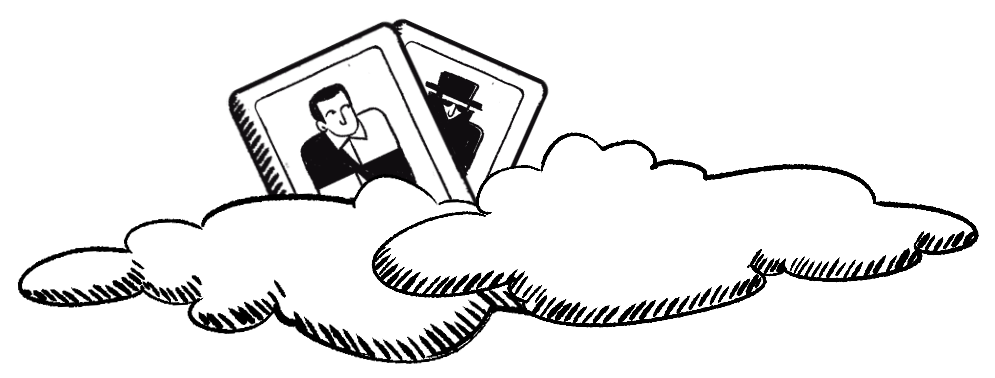
We’re not that different, you and I
That was a long explanation, but now I could finally stop looking for names and start looking at what I can learn about them. Up to this point, a lot of it had been numbers, numbers, numbers. Yes, alright, 0.9
is more similar to something than 0.57
, but it would be nicer to translate that back into a visual.
Look-alike contests are all the rage now, want to have one of our own? $50 cash prize.
We’ll work our way back from the numbers and try to construct what the idea of visual similarity means for people actually look at things together for the first time and trying to make sense of which is which.
Enter any two names
...and see what your face cards look like
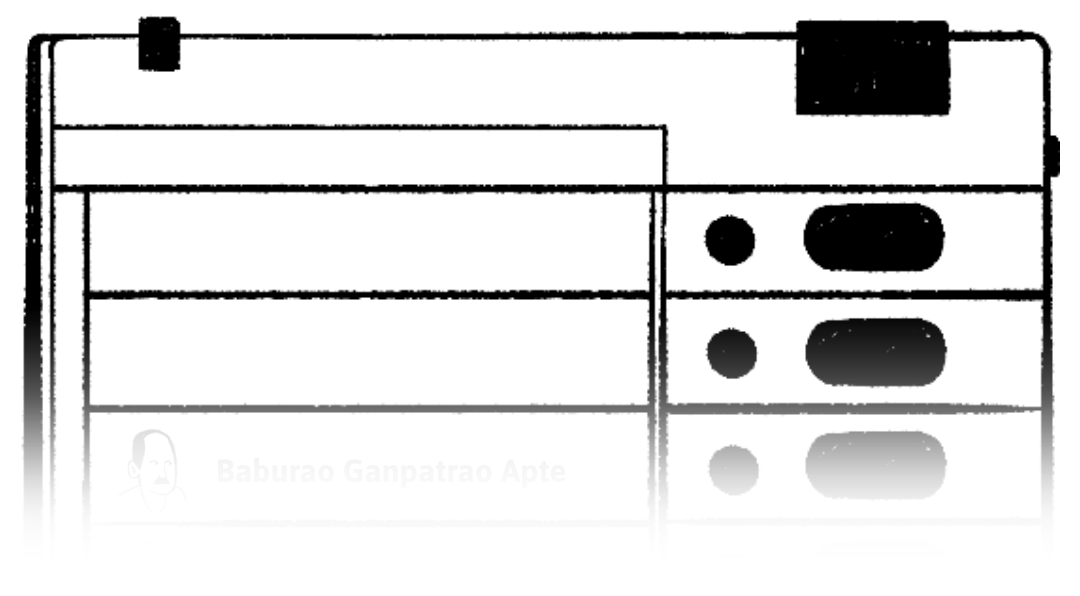
VINOD KUMAR LILAWALI
VINOD KUMAR JORKIYAN
Sufficiently different names are easy to spot, but when they’re not, it takes a bit of work.
I still can’t imagine having to sort between around a dozen Chandu Lal Sahus.
These similar candidates come in various forms. You’ve already seen how algorithms differ based on which state the names are from, so certainly there must be some underlying structure? Well, there is!
So what changed between us?
Certain states are much more represented here than others, but there are still some interesting patterns. First names are more likely to vary a little, and that seems understandable. It’s easier to find someone with a matching last name but a different first name in the same constituency, since surnames often indicate caste/community groups that cluster geographically. For instance, in Punjab, the last name ‘Singh’ usually remains the same and first names are more likely to differ, whereas in Tamil Nadu the trend goes the opposite way. Tamil Nadu also has the most number of cases where only an ‘initial’ change is required – such as the case is with A.K Moorthy’s namesake, K. Moorthy.
Does it ever make a difference?
So far, you’ve seen some ways we can identify and search for namesakes. The question that kept nagging at me was: were there cases where these namesake candidates actually flipped elections?
Most of these candidates barely register in the final tallies - typically getting less than 1% of the vote. Can even small vote shares can matter in some circumstances?
For each pair of similar names, I ran 400 simulations asking a simple question: what if those votes had gone elsewhere?
I gave the simulation different scenarios where varying portions of the namesake candidate’s votes (20%, 40%, 60%, etc.) went to the candidate they shared a name with. These scenarios were weighted based on our similarity scores from the name matching.
While it’s not perfect — voters are complex and elections are messy — it gives us a way to identify the races where namesake candidates might have had their biggest impact.
I narrowed this down to show us examples where the simulation worked in favour of the non-independent candidate 50% of the time.
Elections that could have flipped
Instances with similarly-named candidates and close margins
Year | Election (State/Constituency) | Candidate | Election Margin | Namesake | Similarity |
---|---|---|---|---|---|
2023 | KA Jayanagar | C K Ramamurthy 57,797 votes | 16 | B Ramamurthy 320 votes | 84.5% |
2023 | KA Jayanagar | Sowmya Reddy 57,781 votes | 16 | Sowmya A Reddy 320 votes | 84.5% |
2019 | AP Vijayawada Central | Bonda Umamaheswara Rao 70,696 votes | 25 | Gondesi Umamaheswara Reddy 88 votes | 68.5% |
2019 | AP Vijayawada Central | Malladi Vishnu 70,721 votes | 25 | Malladi Vishnu Vardhan(vishnu) 88 votes | 68.5% |
2005 | BR Mokameh | Anant Kumar Singh 35,877 votes | 94 | Anjani Kumar Sinha 1,141 votes | 73.2% |
2001 | KL Nedumangad | Palode Ravi 62,114 votes | 156 | Vazhode Ravi 442 votes | 68.0% |
2008 | MP Sonkatch | Phool Chand Verma 54,596 votes | 191 | Phoolchand Verma 1,318 votes | 87.6% |
2012 | PB Jagraon | Ishar Singh 52,825 votes | 206 | Avtar Singh 1,192 votes | 65.8% |
2008 | MP Dimani | Shiv Mangal Singh Tomar 24,777 votes | 256 | Shiv Singh Tomar (divakar Giri) 6,340 votes | 77.8% |
2016 | TN Cheyyur | Munusamy A 63,142 votes | 304 | Munusamy S 803 votes | 90.3% |
2022 | UP Bilaspur | Amarjeet Singh 101,691 votes | 307 | Harjeet Singh 702 votes | 81.1% |
2006 | TN Thirumayam | Radhakrishnan M 47,044 votes | 314 | Radhakrishnan A 1,462 votes | 93.6% |
2001 | KL Irinjalakuda | T.sasidharan 53,836 votes | 406 | Sasidharan 1,867 votes | 79.4% |
2020 | BR Bhorey | Jitendra Paswan 73,605 votes | 462 | Jitendra Ram 1,790 votes | 69.2% |
2002 | UP Seorahi | Ramsakal Tiwari 3,845 votes | 465 | Ramdhar Tiwari 4,222 votes | 71.3% |
2006 | KL Pattambi | C.p. Mohammed 57,752 votes | 566 | A.p. Mohamed 1,286 votes | 65.7% |
2006 | KL Pattambi | K.e. Esmail 57,186 votes | 566 | K. Ismail 1,286 votes | 65.7% |
2002 | UK Champawat | Hayat Singh Mahra 7,743 votes | 637 | Madan Singh Mahrana 7,985 votes | 68.4% |
2002 | UK Champawat | Narayan Singh Mahar 2,375 votes | 637 | Madan Singh Mahrana 7,985 votes | 68.4% |
2004 | KA Hosakote | Bachegowda B N 74,973 votes | 835 | Bachegowda A N 2,468 votes | 87.3% |
2004 | KL Alleppey | V M Sudheeran 334,485 votes | 1009 | V S Sudheeran 8,282 votes | 85.7% |
2005 | BR Bhore | Anil Kumar 34,299 votes | 1218 | Anil Kumar Baitha 3,849 votes | 70.1% |
2019 | JH Khunti | Kali Charan Munda 381,193 votes | 1445 | Masih Charan Munda 6,964 votes | 75.3% |
2014 | MH Hingoli | Wankhede Subhash Bapurao 465,765 votes | 1632 | Wankhede Subhash 6,157 votes | 76.3% |
2009 | KL Palakkad | M.b. Rajesh 338,070 votes | 1820 | N.v. Rajesh 5,478 votes | 66.1% |
2009 | KL Palakkad | Satheesan Pacheni 336,250 votes | 1820 | Satheesan. E.v 5,478 votes | 66.1% |
2009 | KA Davanagere | G.m. Siddeswara 423,447 votes | 2024 | G. N. Siddesh 5,694 votes | 82.1% |
2009 | KA Davanagere | S.s. Mallikarjuna 421,423 votes | 2024 | L.s Mallikarjun 5,694 votes | 82.1% |
¹ Table shows examples where the simulation returned a 50% or more probability of the candidate winning
Some of these are incredibly close margins! What was interesting to me was that all of these happened in state assembly elections and not the general elections. Which, I suppose, could make sense? The campaigns in states tend to be more local, and the candidates often don’t get as much attention and press coverage as they do for national races.
To repeat what I said in the beginning, it seems futile to prove, only statistically, that such examples are intentional rather than coincidental. At least we know something about how to look for them now.
Sources and notes
The data on election candidates comes from Trivedi Centre for Political Data. We ran the analysis using R. For the Jaro-Winkler algorithm, we used the stringdist
package. Code for the BI-SIM algorithm was adapted from strcmp2. Dice algorithm was adapted from the dice-coefficient
package available here. This website is built using Sveltekit and uses D3 for visualizations. Website and analysis is available on GitHub.
The artwork has been made available under CC-BY-SA. Find them here.
References
(0) http://www.rochester.edu/college/faculty/alexander_lee/wp-content/uploads/2022/12/paper2.pdf. [link]
(2019) Lok Sabha polls 2019: This election season, it’s all about the names. Hindustan Times. [link]
Ananay Agarwal et al. (2021) TCPD Indian Elections Data v2.0. Trivedi Centre for Political Data, Ashoka University. [link]
Niraj Aswani and Robert Gaizauskas (0) English-Hindi Transliteration using Multiple Similarity Metrics.
Peter Christen (2006) A Comparison of Personal Name Matching: Techniques and Practical Issues. Sixth IEEE International Conference on Data Mining - Workshops (ICDMW'06). doi:10.1109/ICDMW.2006.2
Lynne Emmerton et al. (2020) Development and exploratory analysis of software to detect look-alike, sound-alike medicine names. International Journal of Medical Informatics. doi:10.1016/j.ijmedinf.2020.104119
Grzegorz Kondrak and Bonnie Dorr (0) Identification of Confusable Drug Names: A New Approach and Evaluation Methodology.
Acknowledgements
I am incredibly thankful to Rhea, who came up with the idea of generating faces from numbers. It
is my favorite part of this piece.
A nod to my faulty chair, who finally called off
our year-long fight and let me sit at the proper height while I wrapped this project.
AI Disclosure
No LLM was used for data cleaning, annotation or generating insights. No words were generated with an LLM. The author uses Cursor and Claude as coding assistants.
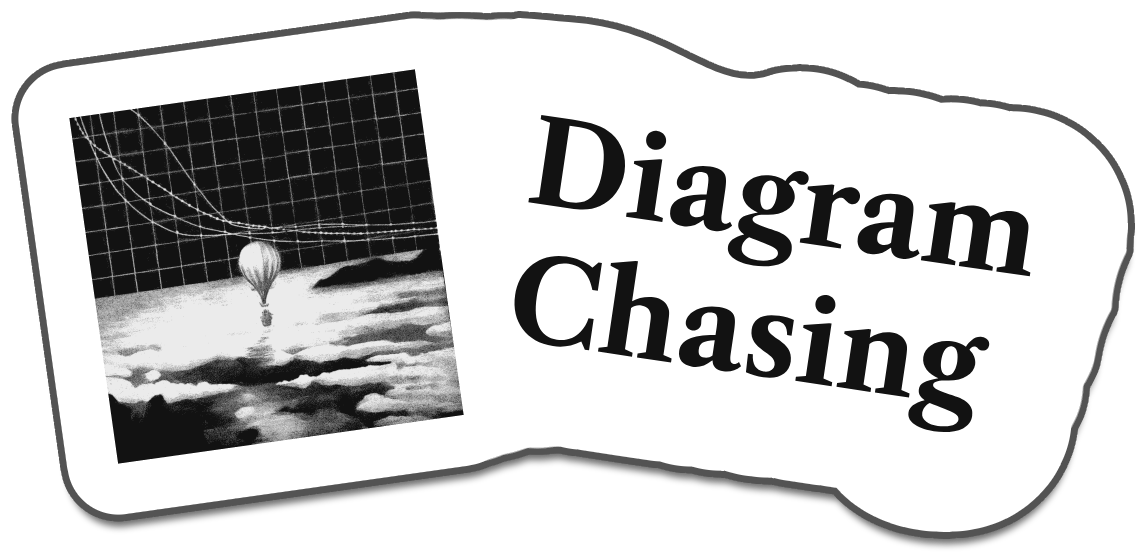